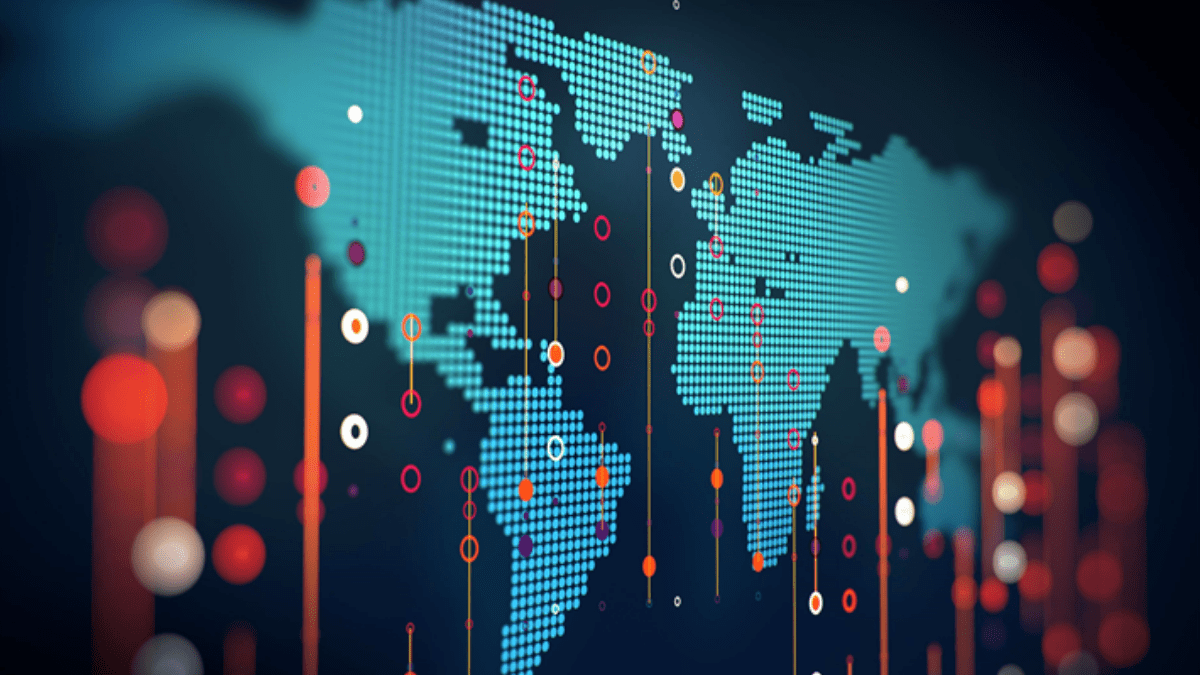
Deep learning, as the fastest-growing field of AI, is enabling significant development in all types of new sectors. Deep learning is already being used to power a variety of applications, including driverless vehicles, smart personal assistants, precision medicine, and much more. Some of the world's top organizations are using AI applications like fraud detection and supply chain efficiency.
Deep learning, as the fastest-growing field of AI, is enabling significant development in all types of new sectors. Deep learning is already being used to power a variety of applications, including driverless vehicles, smart personal assistants, precision medicine, and much more. Some of the world's top organizations are using AI applications like fraud detection and supply chain efficiency.
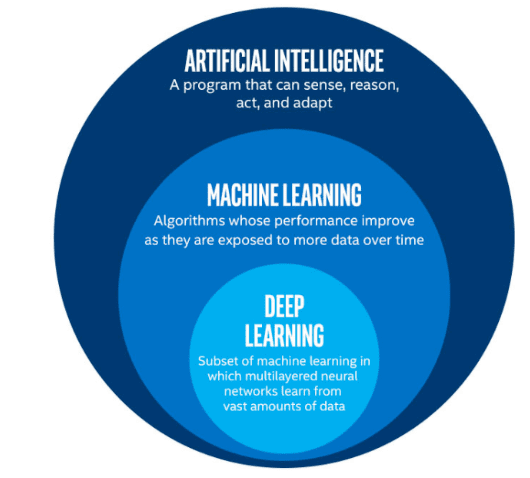
How Deep Learning Works
In the past, analytics has relied on provided data to create new features and variables. While this can provide an accurate, predictive system, it is not very broad. This indicates that the model may not be thorough or accurate enough to handle fresh data. Deep learning algorithms are capable of analyzing and categorizing unstructured data.
What is Deep Learning and how it is used for?
Deep learning is a subset of machine learning that is essentially a three-layer neural network. These neural networks aim to imitate the activity of the human brain by enabling it to "learn" from enormous quantities of data, albeit they fall well short of its capabilities. While a single-layer neural network may produce approximate predictions, more hidden layers can assist to optimize and improve for accuracy.
Many artificial intelligence (AI) apps and services rely on deep learning to increase automation by executing analytical and physical activities without the need for human participation. Everyday goods and services (such as digital assistants, voice-enabled TV remotes, and credit card fraud detection), as well as upcoming innovations, use deep learning technology (such as self-driving cars).
This process has a number of different, useful applications:
Text Classification
Text Classification
Another pillar that deep learning software bears is text classification, which is commonly utilized in combination with other algorithms. It recognizes text as an image. Natural language processing began to evaluate and categorize any documents available on the Internet, assigning them tags and referring them to certain categories. As a result, emailing platforms began to flag emails as spam.
To get fresh insights, developers and marketers collaborate to improve text classification using predictive algorithms. They can now anticipate movie reviews or the feelings expressed in social media posts. Predicting the reactions of bloggers and celebrities is extremely useful for media publishers and any businesses that promote celebrities or bloggers, as it directs them in the right way.
Clustering Information
Clustering is the practice of discovering commonalities in datasets. Unsupervised learning, a kind of deep learning, may perform this on unstructured or unlabeled data. Allowing systems to learn without supervision has the potential to produce extremely accurate models. Clustering can also work in the reverse direction, and filter through massive amounts of data in search of abnormalities or behavior that deviates from the norm.
Image and video enhancement
Deep learning can aid in film creation by automatically synchronizing audio footage with video frames and converting low-resolution photos to high-resolution ones. On pictures and videos, neural networks may also recognize specific products and people. Deep learning can assist surveillance cameras and smart home security systems improve their object detection and facial recognition skills. This will result in higher security product quality and enhanced customer satisfaction.
Language processing
Deep learning is the next generation of machine translation and allows for the automatic translation of the text on pictures as well as the capture and translation of audio. Convolutional neural networks drive immediate visual translation by identifying letters, putting them into words, and constructing phrases out of these words depending on a particular context. NLP (natural language processing) has applications that aren't only limited to text analysis. Spotify trains its algorithms to trawl enormous quantities of data on the internet and detect which terms are used in the context of specific songs or performers. Companies may better understand and engage their consumers and improve their brand image by identifying the tone used by the target audience and feelings associated with brands.
Document parsing
Many businesses are weighed down by massive numbers of paperwork created using various templates. AI-powered automation can aid in the unification and speedy processing of data from multiple papers. At Quantum, we used NLP and machine vision to construct an invoice management solution for a German customer. The technology analyzes papers in seconds, allowing personnel to focus on more analytical and strategic activities.
Fraud detection
Deep learning can be used in a variety of ways to improve customer service, increase efficiency and reduce costs. By 2030, the global market for fraud detection and prevention is estimated to reach $90 billion. Deep learning has the ability to make fraud more predictable and hence avoidable. We'll look at a few intriguing commercial use cases for deep learning in the workplace.
Cybersecurity
Deep learning has a lot of applications in the field of cybersecurity. Deep learning provides a number of advantages over conventional techniques, one of which is accuracy. Because of the significant risk and cost of failing to detect a security attack, the investment in deep learning is warranted. With proper vetting, it's well worth the effort to ensure the time and investment required for implementing a solution.
Social Media
Pinterest's visual search feature uses deep learning to find visually comparable things, colors, patterns, and more. It uses a heavily annotated data set of billions of Pins collected by Pinterest users to teach its system how to detect picture attributes. The characteristics may then be used to choose the best matches by computing a similarity score between any two photos.
Conclusion
Deep learning is a wonderful fit for your business if you require a system that can analyze large volumes of data. Its algorithms can make the most of your data by monitoring and discovering trends quickly, accurately, and at scale. Many sectors, including healthcare, energy, fintech, transportation, and others, are increasingly using deep learning to reimagine old business processes with digital intelligence