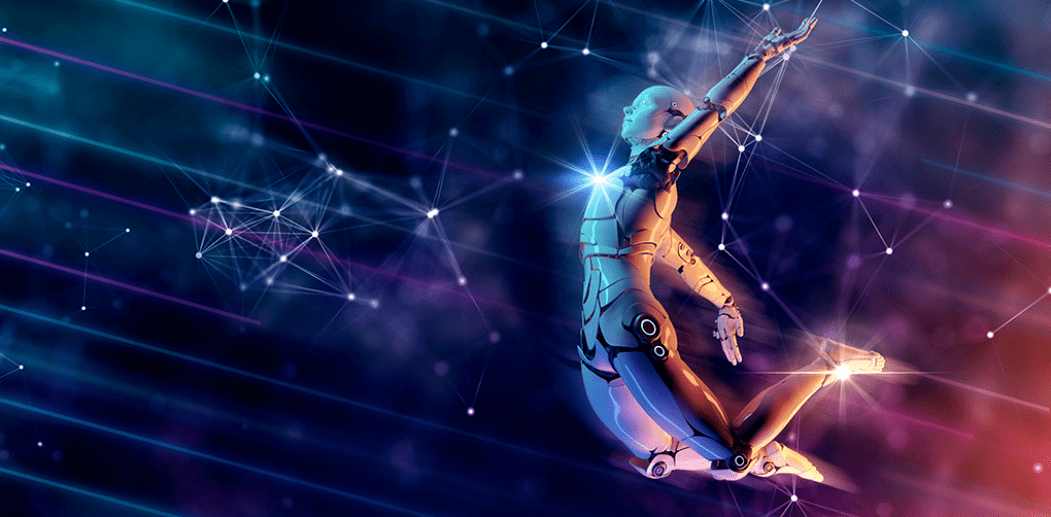
Data-driven decision-making is the practice of using insights derived from the data to make a better decision!
The process of collecting data, transforming it into actionable knowledge, and observing its impact could prove challenging, particularly if your organization has not yet engaged in its data-driven transformation or is not fully equipped to drive it.
Data-driven techniques, ranging from predictive systems to AI-driven automation, are routinely used irregularly throughout organizations.
Creating inefficiencies and putting value on the table. Many business challenges are still solved via traditional methods, which might take months or years. Companies have more tools and skills than ever before, but they aren't always able to turn data into commercial intelligence. Data is seen as a strategic resource, but many organizations have yet to define a data strategy.
Why is it important for a business to be data-driven?
Why is it important for a business to be data-driven?
Data is essential to every Organization’s strategy and serves as a driver for innovation and productivity. Almost every company now invests in data and analytics.
The phrase "data is the new oil" refers to data as a vital resource for enterprises. Data, like oil, can be a tremendously valuable asset provided you know how to extract and utilize it correctly. Raw data has no value on its own.
“Data can be the lifeblood of a company if it is freely exchanged across a whole ecosystem."
A data asset is any data owned by an organization that, when exploited adequately and efficiently, can generate value for the organization.
Data is hard to evaluate, and categorizing "big data" poses further challenges:
- The volume of data to be collected, stored and analyzed.
- The wide variety of data formats and characteristics to manage, as well as the very diverse sources from which it is derived.
- The velocity of data is the speed of both its collection and its changes.
On all three dimensions, the complexity of data managed by businesses has never ceased to increase. Furthermore, the business context represented by this data is changing at a faster rate than ever before.
All of this makes determining the proper area of concentration exceedingly difficult, necessitating the use of standardized processes and frameworks to inventory, appraise, and create value from the data.
Data value involves impacting the business processes, and the 3 challenges:
Data value involves impacting the business processes, and the 3 challenges:
An organization's data assets depend on the usage within an organization, which determines how valuable it is, and ultimately how much it is worth.
Indeed, a data-driven effort is successful when it has an impact on operational processes that are aligned with the company's goals, which necessitates the supplied solution to meet the following three challenges:
- Usable: connected to operational systems and integrated into the technical stack
- Useful: Business users can interpret and act on the results and recommendations if they are well-understood by them.
- Used: Utilized by business users for decision making and continuously improved to keep up with the business context.
The value of data & analytics as a business capability should not be underestimated.
The value of data & analytics as a business capability should not be underestimated.
Implementing the core business processes and functions, achieving measurable impacts, and finally supporting and integrating them...but firms deal with this data-driven transformation — pushing the business past interruptions and proving the worth of data — and it's necessary to put in place a comprehensive value management system to address the problem and reap the rewards.
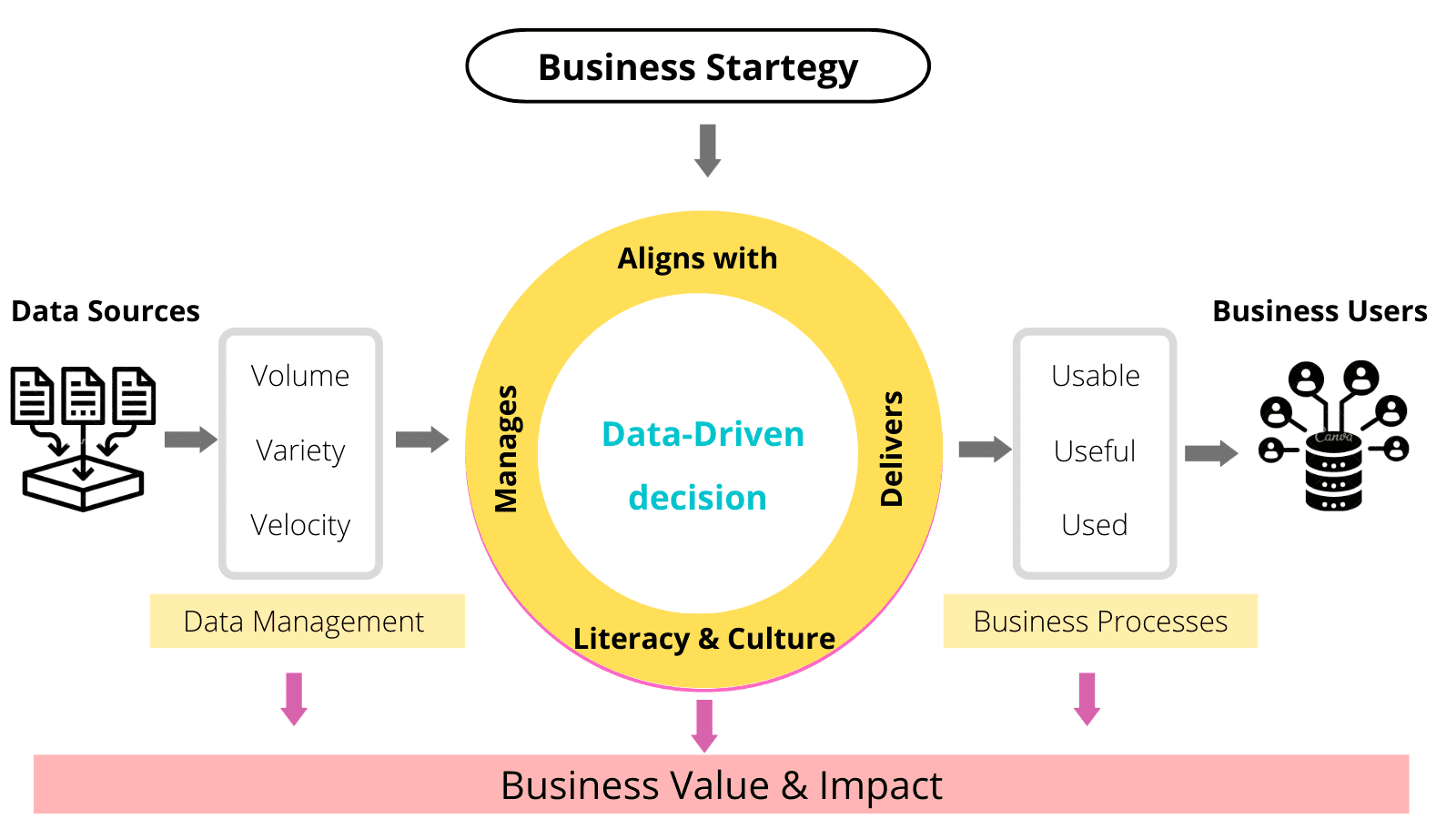
Building a Value-Driven Data Strategy Using a Use Case Approach
Building a Value-Driven Data Strategy Using a Use Case Approach
In a world where nothing ever changes, having a static data strategy probably worked just perfectly. Today, however, your data strategy must be a lifelong process of learning and changing. Your data strategy must be as nimble as the business environment in which you operate.
This necessitates a new approach to developing an organization's data strategy. 9i-oju As a result of this transition, we no longer rely on rigid "big bang" (scale economies) data and analytics methods and instead rely on a value-driven, agile data approach based on continuous, incremental learning and adjustment (economies of learning).
In Part 2 of this blog, I'll explain how we can use data-driven strategies for fundraising and how we can identify relevant fundraising KPIs...