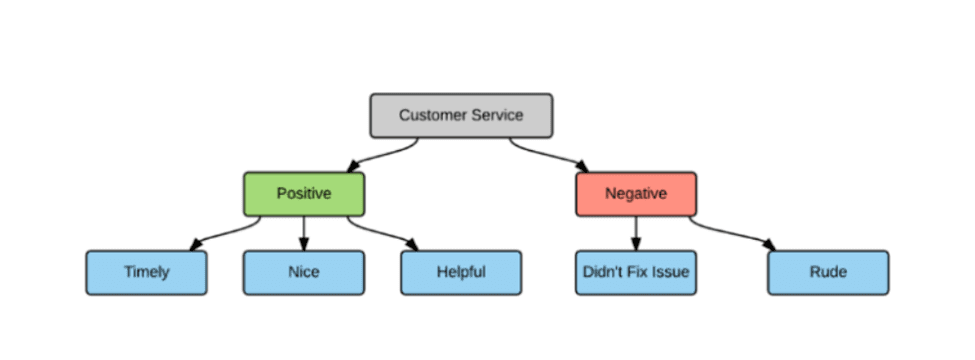
Sentiment analysis (or opinion mining) is a natural language processing (NLP) technique used to determine whether data is positive, negative or neutral. Sentiment analysis is often performed on textual data to help businesses monitor brand and product sentiment in customer feedback, and understand customer needs.
Your company receives hundreds of messages from customers every week. The message could be an account email, a support ticket reply, a live chat message, a tweet, a product review, a voicemail, a comment on a Facebook post, or a web form query... any of these messages could come from any of these sources.
It must be studied, condensed, and, above all, comprehended. The reality is that brands must actively listen to their customers on every channel through which they interact with them, whether it's through phone calls, emails, or live chat. Every brand should prioritize monitoring and analyzing consumer feedback sentiment, but companies have traditionally struggled to handle this data and turn it into meaningful intelligence. That is no longer the case with Sentiment Analysis. Here is what we’re covering in this article:
- What Is Sentiment Analysis?
- Benefits of Sentiment Analysis
- Customer Experience: How to Conduct Sentiment Analysis?
- Sentiment Analysis Challenges
- Personalize Customer Communication
- Conclusion
What Is Sentiment Analysis?
What Is Sentiment Analysis?
A simple definition of sentiment analysis is the measurement of how customers respond to a product, service, or brand. Sentiment analysis, a tool based on natural language processing and text analysis, is used today in many ways, most commonly to analyze customer comments and feedback. For example, some tools are available to analyze comments made on social media or customer feedback channels to determine whether they are positive, negative, or neutral. After that, enterprises can apply the insights gained to improve products, services, Web sites, customer communications, marketing campaigns, etc.
Benefits of Sentiment Analysis
Benefits of Sentiment Analysis
Prospects for upselling:
It allows you to segment your entire customer base into different segments, such as happiest customers, best buyers, frustrated customers, and customers about to leave your brand. By taking advantage of your happy customers, you can increase upselling opportunities.
Exceptional customer support
The sentiment analysis can be used to train your chatbots to detect when to escalate the chat to an agent or route it to experienced professionals.You can improve customer service and deliver a delightful experience this way.
Triggers of Emotions
Most customer actions are influenced by their emotions and the experiences they have. It is important to provide excellent customer service because it triggers positive emotions, which lead to positive behaviors. In contrast, poor customer service can increase negative feelings and actions.
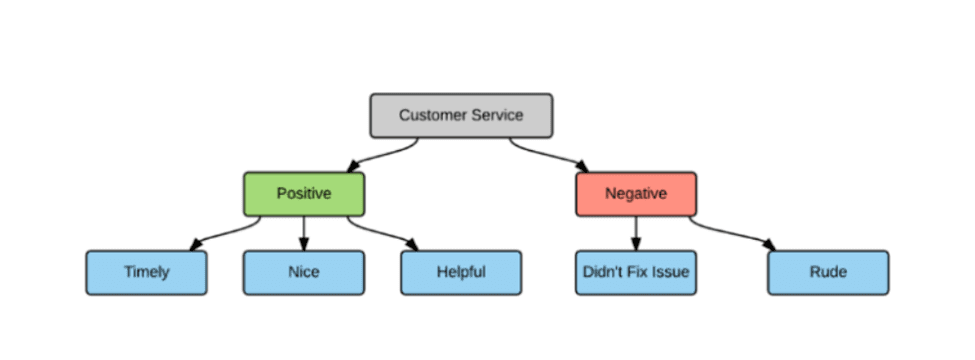
You can use sentiment analysis to figure out which messages and chats elicit emotional responses from your customers.
For example, Customers may be irritated by the phrase "We will get back to you in some time,” Customers respond positively when you use emoticons in a chat, message, or email.
Customers' emotions and actions are decoded via sentiment analysis. You may use this data to make informed decisions about how to improve the quality of your customer service.
Some other benefits of sentiment analysis are:
- Tackle customer churn
- Monitor customer satisfaction
- Seamless escalation of customer issues
- Insights-driven marketing strategies and campaigns
- Improved customer experience
Customer Experience: How to Conduct Sentiment Analysis?
Customer Experience: How to Conduct Sentiment Analysis?
Customer sentiment can be collected in a number of ways and analyzed for business purposes. The following sections discuss different approaches to sentiment analysis online.
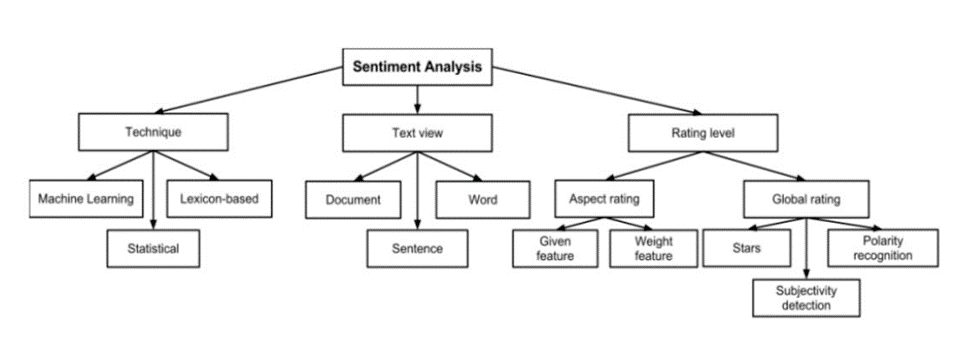
1. Top-level document sentiment analysis:
The tone of a document is determined via sentiment analysis at the document level. It features data processing workflows that involve analyzing the tone of a text block to determine if it is negative, positive, or neutral. Some tools additionally provide a NULL result, which indicates that the text is neither negative nor positive.
When the text to be evaluated is lengthy, this sentiment analysis method performs best.
While top-level sentiment research can provide an overview of brand impression within customer groups, it cannot deliver practical deeper insights.
Take a look at the reviews below, for example. These are some excellent examples of sentiment analysis:
Jack: This brand is terrible...poorest customer service ever!
Brody: I don’t like their products…Such poor quality!!
While a top-level sentiment analysis will reveal that both of these statements are negative, it will not reveal the reasons behind these negative reactions.
As a result, if you want to analyze overall brand perception, the top document-level analysis is the way to go.
2. Paragraph-level analysis of sentiment:
Since sentiment analysis is performed at the paragraph level, it is more sophisticated. As a result, the processor goes over each text paragraph to see if it has a negative or positive tone, both tones, or none at all.
It's ideal for delving into big paragraphs and long text documents with various sections. Blogs, critic reviews, and in-depth reviews are all excellent examples.
The ability of modern clients to consume content is astounding. As a result, blogs remain popular among the general public. This necessitates the processing of blogs or other written items with lengthy paragraphs. You can assess the tone of paragraphs to determine whether the content will be a success or a failure with clients.
3. Text-level analysis of sentiment:
The total number of people using social media is 4.14 billion. This makes it a profitable platform for finding clients, tracking their activity, and interacting with prospects.
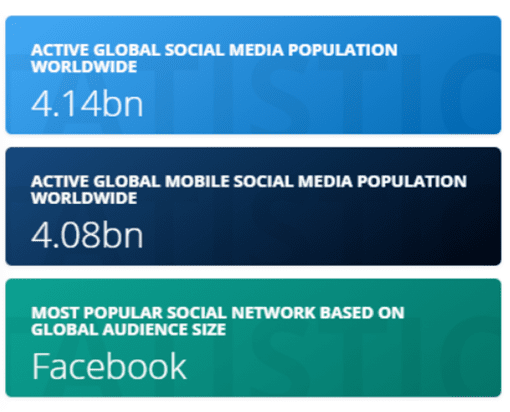
Social media is a massive data storehouse and one of the most popular communication avenues for your customers to ask questions, share their experiences and opinions, and seek help.
You may mine this business data and extract numerous sorts of client sentiments using a clever and straightforward social media monitoring platform.
You can get useful information about how people perceive your brand and how they react to your marketing activities. Direct and passive social media mentions can also be used to acquire important client feedback.
Sentiment Analysis Challenges
Sentiment Analysis Challenges
Natural language processing's sentiment analysis is one of the hardest tasks, as even humans struggle to analyze emotions accurately.
Although data scientists are improving their ability to create more accurate sentiment classifiers, they still have a long way to go. Let's look at some of the biggest issues with machine-based sentiment analysis:
1. Subjectivity and Tone
Subjective and objective texts are the two sorts of texts. Subjective texts express sentiments, while objective ones do not. Let's say you want to examine the sentiment of the following two texts:
The package is attractive.
The package is crimson in color.
Most people would argue that the first one is positive and the second one is neutral, right? When it comes to creating sentiment, all predicates (adjectives, verbs, and some nouns) should not be treated the same. Nice is more subjective than red in the instances above.
2. Irony and Sarcasm
People convey their negative attitudes using positive phrases in irony and sarcasm, which can be difficult for robots to discern without a detailed understanding of the situation in which a feeling was expressed.
Consider some possible responses to the question, "Did you enjoy your shopping experience with us?"
Sure, go ahead. So silky!
There are several!
What emotion do you think the replies above convey? Isn't it possible that the first exclamation mark reaction is negative? The issue is that there is no textual cue that will assist a computer to understand, or at the very least question, that feeling, because yes and certainly are frequently used in positive or neutral messages.
What about the second option? Although the sentiment is positive in this context, we're sure you can think of a number of other situations in which the identical reaction could communicate negative emotion.
3. Comparisons
Another difficulty worth tackling in sentiment analysis is how to treat comparisons. Examine the following passages:
This is a fantastic product.
This is far superior to the previous software.
It's preferable to doing nothing.
To be accurately identified, the initial comparison does not require any contextual clues. It is obvious that it is good.
However, classifying the second and third texts is more difficult. Are they neutral, good, or even negative? Context is important once more. If the 'earlier tools' in the second text are deemed useless, the second text resembles the third text.
4. Emojis
According to Guibon et al., there are two sorts of emojis. Western emojis (for example, D) are only one or two characters long, whereas Eastern emojis (for example, () / ) are a lengthier vertical combination of characters. Emojis play a significant role in conveying emotion in language, especially in tweets.
When performing sentiment analysis on tweets, you should pay close attention to both character and word levels. It's possible that a lot of preprocessing is required. To aid boost sentiment analysis performance, you could want to preprocess social media information and convert both Western and Eastern emojis into tokens, then whitelist them (i.e. always treat them as a feature for classification reasons).
This is a rather complete list of emojis and their meanings.
Personalize Customer Communication
Sentiment Analysis can decode a customer's emotions, allowing you to customize the engagement and boost customer satisfaction. Customers expect organizations to send them individualized messaging. They want the company to understand exactly what the customer wants and feels, and to provide meaningful experiences that are suited to their requirements.Sentiment Analysis can also determine which kind of messaging causes clients to have negative or good emotions.
Agents can tailor polite responses in both scenarios to positively touch customers' emotions and leave them with a positive experience.Agents can tailor polite responses in both scenarios to positively touch customers' emotions and leave them with a positive experience.Sentiment Analysis can also determine which kind of messaging causes clients to have negative or good emotions.Agents can tailor polite responses in both scenarios to positively touch customers' emotions and leave them with a positive experience.
Conclusion
Sentiment Analysis allows companies to properly comprehend how a customer feels about their brand and their interactions with it. It gathers data from all around the internet to help you provide an amazing client experience.
Sentiment Analysis offers the road for you to address any potential issues that can irritate your customers and reach out to them to provide a pleasant experience. The text-mining tool recognizes emotional triggers and assists you in optimizing your customer service to keep loyal consumers engaged.