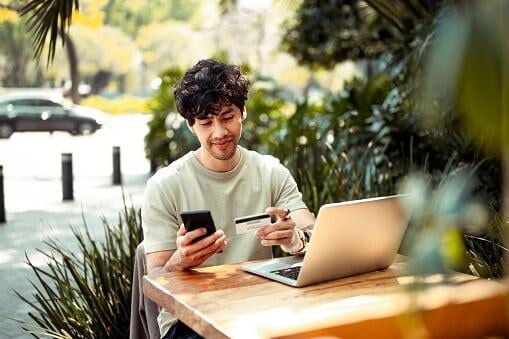
Retail goes hand in hand with machine learning to forecast market trends and pricing, personalize customers’ experience, and keep consumers engaged.
The retail industry continues to progress in terms of how they leverage machine learning to generate data and identify the current market trends and customer sentiments. Using machine learning has allowed those in retail to gain better foresight on how to approach, engage, and keep customers satisfied, which would, in turn, improve sales and give retailers a competitive edge.
Machine learning has been defined as a method in computer science that focuses on the use of data and algorithms to become more accurate at predicting outcomes while continuously learning, improving, and adapting to new data without human intervention.
Nowadays, retail goes hand in hand with machine learning in order to regularly forecast market trends and pricing, personalize customers’ experience, and keep loyal as well as prospective consumers engaged.
The benefits of machine learning in retail
The benefits of machine learning in retail
There are several benefits of machine learning in retail, among the top uses are the following:
- Personalized Recommendations
- Forecasted Pricing
- Tuning in with customers
- Responsive AI-Chatbots and Virtual Assistants
- Curated Social Media Reach
Top use cases of data science in retail
Personalized Recommendations
Personalized Recommendations
Recommenders system is one of the most common uses of machine learning in retail with how it analyzes customer’s purchasing patterns and uses that data in order to personalize and suggest relevant items that the said customer may be interested in buying.
By keeping note of what customers want and providing them with recommendations fit for what they’re searching for, retailers would be able to form a more solid connection with their customers wherein they wouldn't have to use other platforms for their shopping needs.
Amazon.com uses a recommender system called ‘item-to-item collaborative filtering’ on most pages of their websites and e-mail campaign. This method finds the similarities between several pairs of items that have been rated by the user and thereafter generates a list of recommendations for them. Retailers can easily adopt this approach as well in their businesses.
Forecasted Pricing
Forecasted Pricing
Aside from the seasonal change in prices of products, retailers can change the prices of their items according to different factors such as demand, supply, competition, brand, and customer sentiment of pricing variables of different products and services.
Through its process of using collected data to predict the most valuable predictions and results, predictive analytics in machine learning can significantly help in figuring out what the best price for specific products and services would be.
Setting the prices according to what’s been forecasted through the predictive analytics approach, retailers can take this chance to offer promotions and discounts to customers which would drive an increase in sales.
Tuning in with customers
Tuning in with customers
Another use of predictive analytics would be in understanding customer behavior. By using the data collected from customers’ behaviors in their past purchasing or shopping experiences, retailers can adapt their marketing efforts according to what their customers are more likely to purchase.
An example of this is how Netflix uses machine learning to understand how its users consume television and film content. The data collected translates into informed strategic decisions such as predicting and offering users well-matched recommendations based on what they’ve watched or those related to it.
Responsive AI-Chatbots and Virtual Assistants
Responsive AI-Chatbots and Virtual Assistants
North Face, an outdoor clothing retailer, uses AI and machine learning to offer users a highly personalized shopping experience through “IBM Watson” that allows shoppers to interact with a virtual assistant that walks users through a series of questions and learn from their answers to offer relevant products according to their preferences.
Curated Social Media Reach
Curated Social Media Reach
Social media has widened its role in the retail industry with how it can reach a vast amount of people on different platforms. It’s no surprise to see retailers establishing and keeping an active presence online. From browsing through retailers’ products to contacting retailers o their page, social media is used in various ways to understand and learn more about customer sentiment.
These online platforms are used to monitor customer sentiments and find out what topics or trends drive traffic and engagement among their customers. Retailers can also generate social media content curated to relevant market trends.
Data science, or machine learning in particular, has elevated the retail industry to address challenges and generate convenient means to understand, learn, and adapt to customers’ needs and behavior. Over here we provided a brief overview of some of the important use cases in the Retail Industry for Data Science.
There could be numerous other use cases aside from what we covered. Each of these use cases could get very complex depending upon several factors such as the amount of data and type of data, among others. Reach out to One Connect Solutions if you’re looking to implement or enable these machine learning uses in your organization.