Predictive analytics has revolutionized the way businesses and organizations leverage data to make informed decisions and gain a competitive edge. By analyzing historical data, identifying patterns, and predicting future trends, predictive analytics models enable businesses to optimize operations, enhance customer experiences, and drive growth. In this article, we will explore the benefits of predictive analytics in different business and organization models, highlighting the key types of predictive analytics models and their applications.
Understanding Predictive Analytics
Understanding Predictive Analytics
Predictive analytics is a branch of data analytics that uses historical data to forecast future outcomes and trends. It involves applying statistical algorithms and machine learning techniques to analyze patterns and relationships within datasets. By identifying these patterns, predictive analytics models can make accurate predictions and help businesses make informed decisions.
The machine learning lifecycle consists of three main stages: Preparation, Modeling, and Deployment. Each stage is comprised of several steps that collectively optimize the process of developing and implementing predictive models.
Data Preparation
Data preparation is a crucial step in the machine learning lifecycle as it accounts for a significant portion of a data scientist's work. This stage involves acquiring, cleaning, and labeling data, as well as performing feature engineering to enhance the dataset. SQL, a powerful tool for data transformation, is commonly used for feature engineering, as it allows data scientists to manipulate and enrich datasets by applying various techniques.
Modeling
In the modeling stage, data scientists select the appropriate predictive model based on the nature of the problem and the available data. Classification models, one type of predictive model, categorize data into groups based on historical data. Clustering models, on the other hand, group similar data points together based on common attributes. Time series models analyze data collected over time to identify patterns and predict future trends.
Deployment
Once the predictive model is developed, it needs to be deployed in a production environment to generate actionable insights. This involves integrating the model into existing systems, monitoring its performance, and continuously updating it as new data becomes available. The deployment stage ensures that the predictive model is providing accurate and up-to-date predictions, enabling businesses to make data-driven decisions.Types of Predictive Analytics Models
Types of Predictive Analytics Models
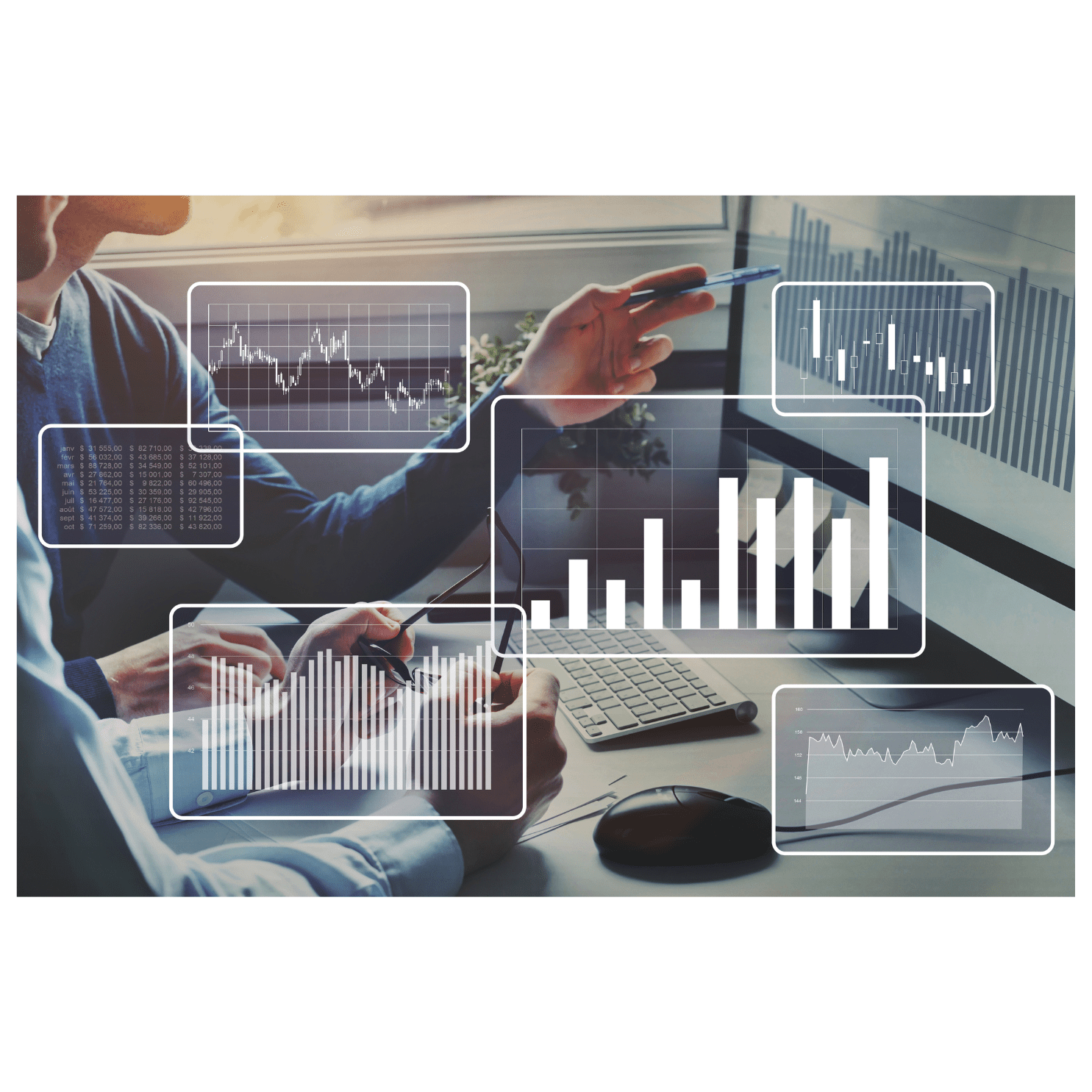
Classification Models Classification models are a type of supervised machine learning models that categorize data based on historical data and relationships within a given dataset. These models are widely used for segmentation purposes, customer profiling, fraud detection, and credit risk evaluation. Popular classification models include logistic regression, decision trees, random forest, neural networks, and Naive Bayes.
Classification models enable businesses to classify customers or prospects into groups based on specific criteria, allowing for targeted marketing strategies, personalized recommendations, and improved customer experiences. By accurately predicting customer behavior, businesses can optimize their marketing efforts, reduce churn rates, and maximize customer lifetime value.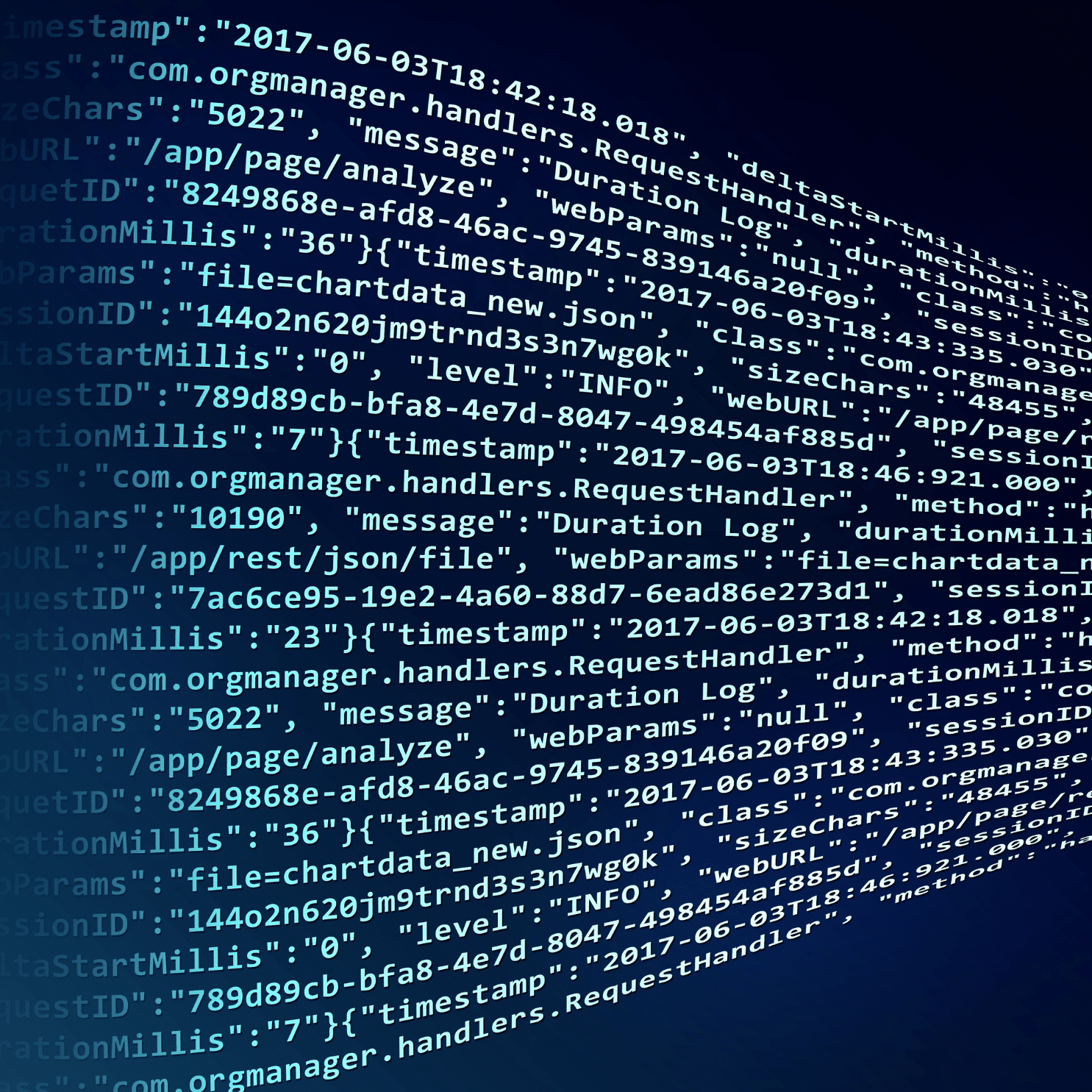
Clustering Models
Clustering models, on the other hand, fall under the category of unsupervised machine learning models. These models group data based on similarities in attributes, allowing businesses to identify patterns and segments within their datasets. Clustering models are particularly useful for customer segmentation, market research, anomaly detection, and recommendation systems.
By clustering customers into distinct groups based on their preferences, behaviors, or characteristics, businesses can tailor their products, services, and marketing campaigns to specific customer segments. This leads to improved customer targeting, higher customer satisfaction, and increased sales.Common clustering algorithms include k-means clustering, mean-shift clustering, DBSCAN, EM clustering using GMM, and hierarchical clustering. Each algorithm has its own strengths and weaknesses, making it crucial for businesses to select the most suitable algorithm based on their specific needs and dataset characteristics.
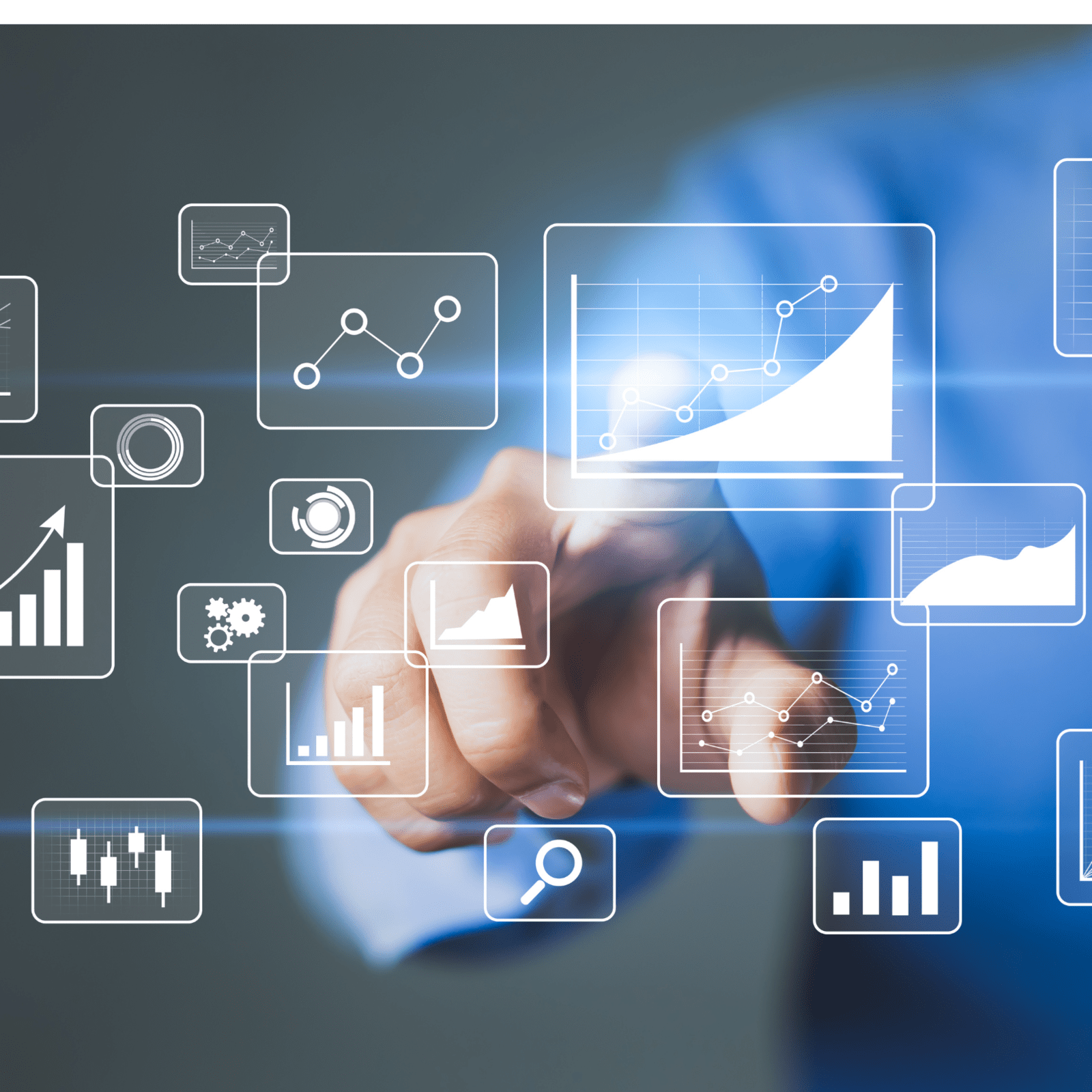
Time Series Models
Time series models focus on analyzing data that is collected over time at regular intervals, such as daily, weekly, or monthly. These models are used to identify trends, seasonality, and cyclical patterns in data, enabling businesses to make accurate forecasts and predictions. Time series models are widely used in industries such as finance, supply chain management, and call center operations.
By analyzing historical data, time series models can predict future values and behaviors, allowing businesses to make informed decisions and plan for future demand, resource allocation, and operational efficiency. Commonly used time series models include autoregressive (AR), moving average (MA), ARMA, and ARIMA models.
Time series models are particularly valuable in industries where demand forecasting, inventory management, and resource planning are critical. By accurately predicting future demand or customer behavior, businesses can optimize their operations, reduce costs, and avoid stockouts or overstock situations.
How is this beneficial?
One of the primary benefits of predictive analytics is its ability to enhance decision-making. By analyzing historical data, identifying patterns, and making accurate predictions, businesses can make informed decisions and take proactive actions. Whether it's optimizing inventory levels, identifying potential risks, or predicting customer churn, predictive analytics provides valuable insights that guide decision-making processes.With predictive analytics, businesses can reduce the reliance on intuition and guesswork, instead relying on data-driven insights. This leads to more confident decision-making, reduced risks, and improved overall business performance.
Predictive analytics allows businesses to gain a deeper understanding of their customers, their preferences, and their behaviors. By analyzing customer data, businesses can identify patterns and trends, enabling personalized marketing campaigns
Predictive analytics is a game-changer for businesses and organizations looking to gain a competitive advantage in today's data-driven world. By leveraging machine learning models and powerful databases like ClickHouse and Pecan, organizations can extract valuable insights from historical data, make accurate predictions, and make informed decisions. The integration of predictive capabilities with databases streamlines the machine learning lifecycle and empowers data analysts to generate actionable insights. With predictive analytics, businesses can stay ahead of the competition, optimize operations, and drive growth in an increasingly data-centric landscape.